The new AI is able to predict when and why protein aggregation occurs, a mechanism linked to Alzheimer’s and 50 other diseases that affect 500 million people. The results show great potential for research into neurodegenerative diseases and for improving drug production, reducing costs and increasing efficiency. The study, published today in Science Avances, is the result of a collaboration between the Centre for Genomic Regulation (CRG) and the Institute of Bioengineering of Catalonia (IBEC).
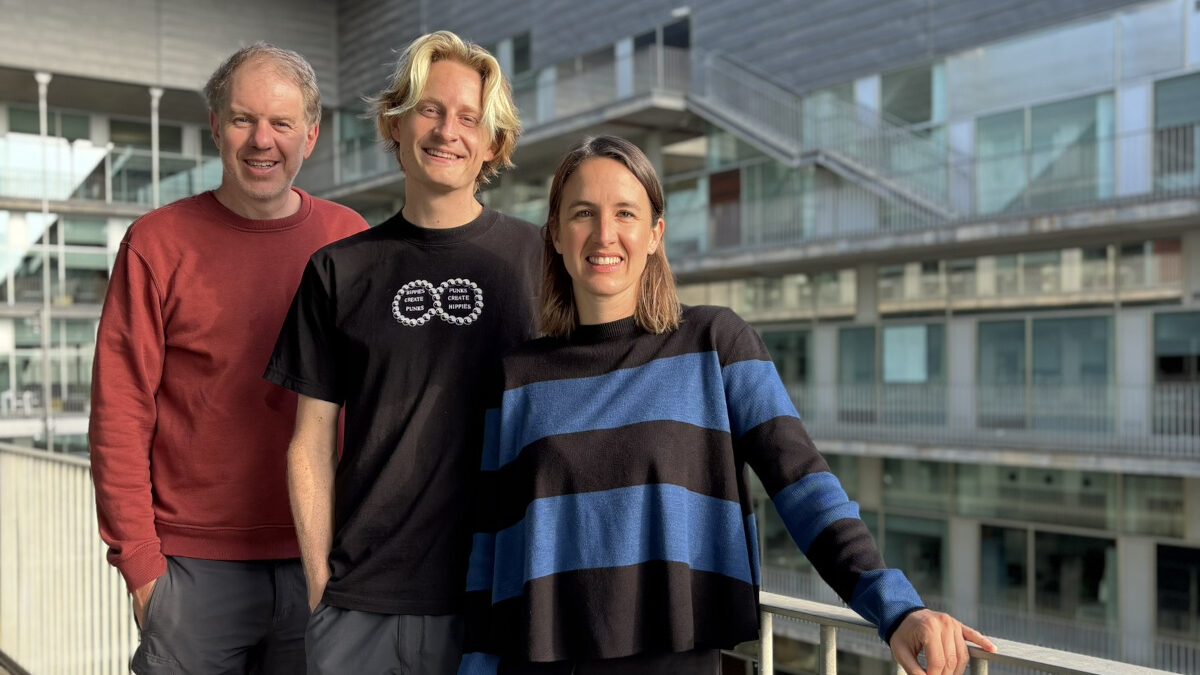
An AI tool has made a step forward in translating the language proteins use to dictate whether they form sticky clumps similar to those linked to Alzheimer’s Disease and around fifty other types of human disease. In a departure from typical “black-box” AI models, the new tool, CANYA, was designed to be able to explain its decisions, revealing the specific chemical patterns that drive or prevent harmful protein folding.
The discovery, published today in the journal Science Advances, was possible thanks to the largest-ever dataset on protein aggregation created to date. The study gives new insights about the molecular mechanisms underpinning sticky proteins, which are linked to diseases affecting half a billion people worldwide.
Protein clumping, or amyloid aggregation, is a health hazard that disrupts normal cell function. When certain patches in proteins stick to each other, proteins grow into dense fibrous masses that have pathological consequences.
Protein aggregation is a major headache for pharmaceutical companies. CANYA can help guide efforts to engineer antibodies and enzymes that are less likely to stick together and reduce expensive setbacks in the process.
Benedetta Bolognesi
While the study has some implications for accelerating research efforts for neurodegenerative diseases, it’s more immediate impact will be in biotechnology. Many drugs are proteins, and they are often hampered by unwanted clumping.
“Protein aggregation is a major headache for pharmaceutical companies,” says Dr. Benedetta Bolognesi, co-corresponding author of the study and Group Leader at the Institute for Bioengineering of Catalonia (IBEC).
“If a therapeutic protein starts aggregating, manufacturing batches can fail, costing time and money. CANYA can help guide efforts to engineer antibodies and enzymes that are less likely to stick together and reduce expensive setbacks in the process,” she adds.
Protein clumps are formed using a poorly understood language. Proteins are made of twenty different types of amino acids. Instead of the usual A, C, G, T letters that make up the language of DNA, a protein’s language has twenty different letters, different combinations of which form “words” or “motifs”.
Researchers have long sought to decipher which combinations of motifs cause clumping and which others enable proteins to fold without error. Artificial intelligence tools that treat amino acids like the alphabet of a mysterious language could help identify the precise words or motifs responsible, but the quality and volume of data about protein aggregation needed to feed models have been historically scant or restricted to very small protein fragments.
The study addressed this challenge by carrying out large-scale experiments. The authors of the study created over 100,000 completely random protein fragments, each 20 amino acids long, from scratch. The ability for each synthetic fragment to clump was tested in living yeast cells. If a particular fragment triggered clump formation, the yeast cells would grow in a certain way that could be measured by the researchers to determine cause and effect.
We created truly random protein fragments including many versions not found in nature, providing lots of data points to help understand more general laws of aggregation behaviour.
Mike Thompson
Around one in every five protein fragments (21,936/100,000) caused clumping, while the rest did not. While previous studies might have tracked a handful sequences, the new dataset captures a much bigger catalogue of the different protein variants which can cause amyloid aggregation.
“We created truly random protein fragments including many versions not found in nature. Evolution has explored only a fraction of all possible protein sequences, while our approach helps us peer into a much bigger galaxy of possibilities, providing lots of data points to help understand more general laws of aggregation behaviour,” explains Dr. Mike Thompson, first author of the study and postdoctoral researcher at the Centre for Genomic Regulation (CRG).
The vast amount of data generated from the experiments was used to train CANYA. The researchers decided to create it using the principles of “explainable AI”, making its decision-making processes transparent and understandable to humans. This meant sacrificing a little bit of its predictive power, which is usually higher in “black-box” AIs. Despite this, CANYA proved to be around 15% more accurate than existing models.
Specifically, CANYA is a convolution-attention model, a hybrid tool borrowing from two distinct corners of AI. Convolution models, like those used in image recognition, scan photos for features like an ear or a nose to identify a face, except in this case CANYA skims through the protein chain to find meaningful features like motifs or “words”.
Attention AI models are used by language translation tools to identify key phrases in a sentence before deciding on the best translation. The researchers incorporated this technique to help CANYA figure out which motifs matter most in the grand scheme of the entire protein.
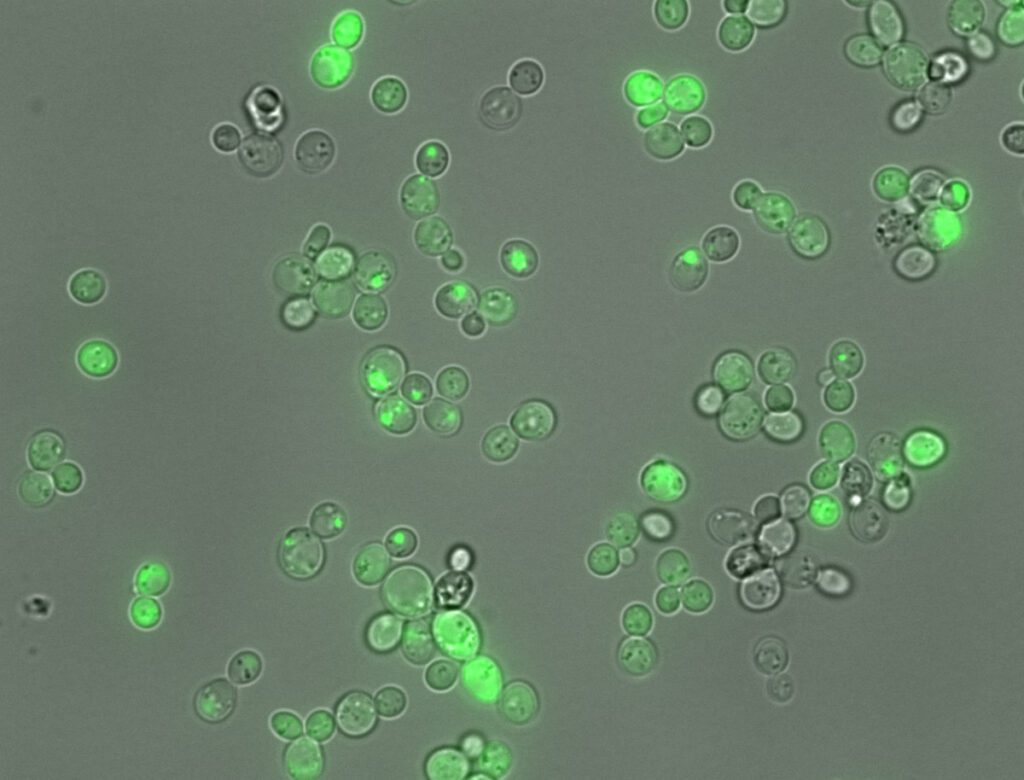
Together, these two approaches help CANYA see local motifs up close while also spotting their bigger-picture importance. The researchers could use this information to not just predict which motifs in the protein chain encourage clumping, block it, or something in between, but also understand why.
For example, CANYA showed that small pockets of water-repelling amino acids are more likely to spark clumping, while some motifs have a bigger impact on clumping if they’re near the start of a protein sequence rather than at the end. The observations align with previous findings researchers have seen under the microscope in known amyloid fibrils.
But CANYA also found new rules driving protein aggregation. For instance, certain building blocks of proteins, so-called charged amino acids, are normally thought to prevent clumping. But it turns out that in the context of other specific building blocks, they can actually promote clumping.
In its current form, CANYA primarily explains protein aggregation in yes or no terms, i.e. it works as a so-called “classifier”. The researchers next want to refine the system so it can predict and compare aggregation speeds rather than just aggregation likelihood. This could help predict which protein variants form clumps quickly and which do so more slowly, a vital factor in neurodegenerative diseases where the timing of amyloid formation matters just as much as the fact that it happens at all.
This project is a great example of how combining large-scale data generation with AI can accelerate research. It’s also a very cost-effective method to generate data.
Ben Lehner
“There are 1024 quintillion ways of creating a protein fragment that is 20-amino acids long. So far, we’ve trained an AI with just 100,000 fragments. We want to improve it by making more and bigger fragments. This is just the first step but our work shows it is possible to decipher the language of protein aggregation. This is incredibly important for our understanding of human disease but also to guide synthetic biology efforts” concludes Dr. Bolognesi.
“This project is a great example of how combining large-scale data generation with AI can accelerate research. It’s also a very cost-effective method to generate data,” says ICREA Research Professor Ben Lehner, co-corresponding author and Group Leader at the Centre for Genomic Regulation (CRG) and the Wellcome Sanger Institute.
“Using DNA synthesis and sequencing we can perform hundreds of thousands of experiments in a single tube, generating the data we need to train AI models. This is an approach we are applying to many difficult problems in biology. The goal is to make biology predictable and programmable,” he adds.
The study is a joint collaborative effort by ICREA Research Professor Ben Lehner’s lab at the Centre for Genomic Regulation (CRG) and Benedetta Bolognesi’s lab at the Institute for Bioengineering of Catalonia (IBEC). Researchers from Cold Spring Harbor Laboratory (CSHL) and Wellcome Sanger Institute also collaborated in the study. It was funded by ”La Caixa” Research Foundation, the European Research Council and the Spanish Ministry of Science and Innovation.
Reference article
Mike Thompson, Mariano Martín, Trinidad Sanmartín Olmo, Chandana Rajesh, Peter K. Koo, Benedetta Bolognesi, Ben Lehner. Massive experimental quantification allows interpretable deep learning of protein aggregation. Science Advances (2025). DOI: 10.1126/sciadv.adt5111