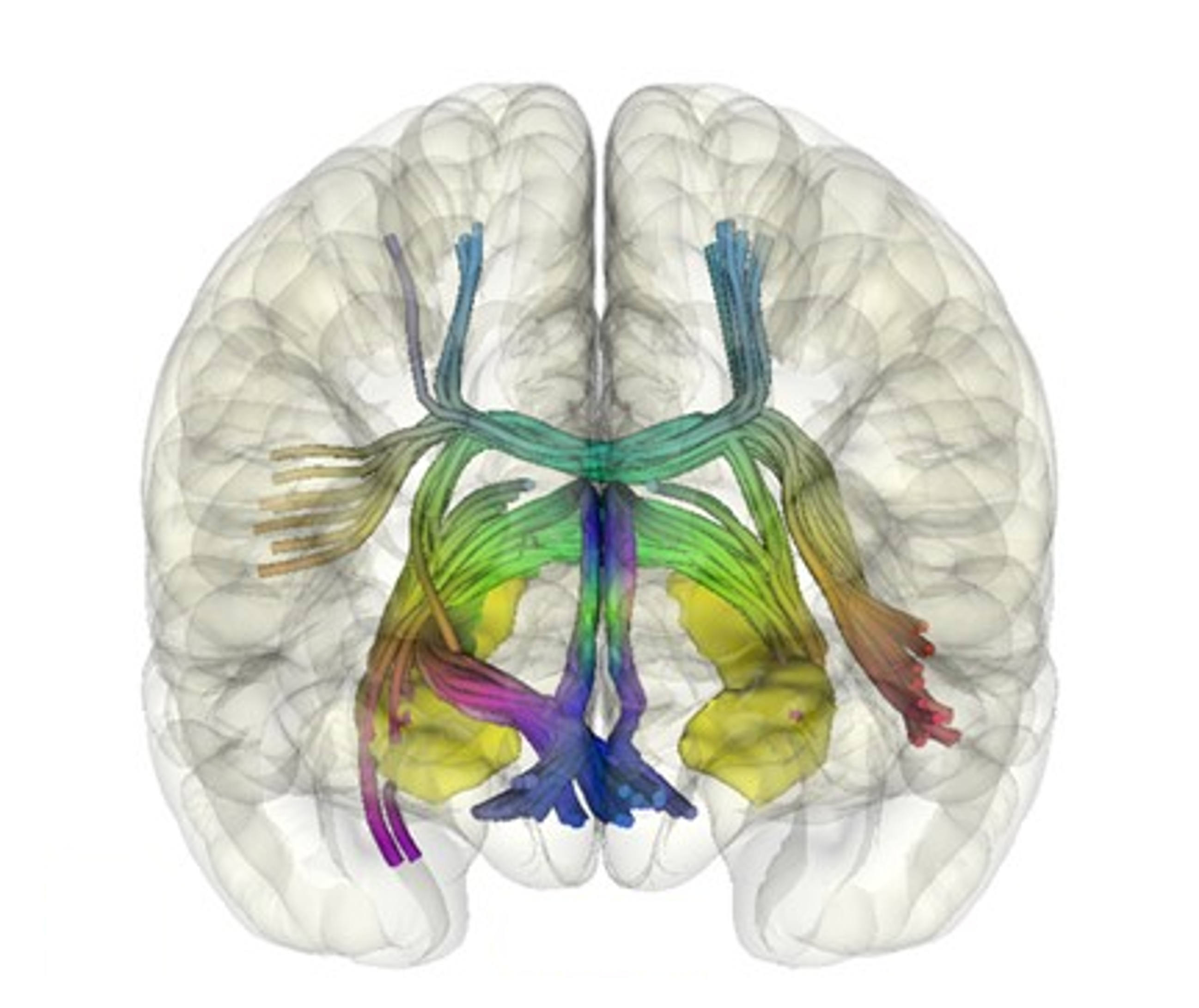
Thanks to the so-called deep learning, a subset of artificial intelligence (AI) algorithms inspired by the brain, machines can match human performance in perception and language recognition and even outperform humans in certain tasks. But do these synthetic biologically inspired systems learn in the same way that we do?
According to a new article by first author Dr. Diogo Santos–Pata from the Synthetic Perceptive, Emotive and Cognitive Systems lab (SPECS) at IBEC led by ICREA Professor Paul Verschure, in collaboration with Prof. Ivan Soltesz at Stanford University, the mechanism of autonomous learning underlying these AI systems reflects nature more closely than previously thought. With their hypothesis and model, these scientists offer new insights into how we learn and store memories.
The work, published in the prestigious scientific journal Trends in Cognitive Sciences, is relevant for improving memory deficits in humans and for building new and advanced forms of artificial memory systems.
Learning without a teacher
“The brain is considered an autonomous learning system”. In other words, it can detect patterns and acquire new knowledge without external guidance. Until recently, this was not the case for AI — any data being fed to machine learning systems needed to be tagged first. This so-called symbol grounding problem hampers progress in AI for the last decades. Paul Verschure and colleagues have systematically addressed the ability of cognitive systems to autonomously acquire knowledge, or so-called epistemic autonomy.
Image: owned by SPECS-lab (Shutterstock)
We resolved two riddles which appeared unrelated but are intertwined: that epistemic autonomy of the brain is based on its ability to set self-generated learning objectives and that inhibitory signals are propagated through the brain to improve learning.
Paul Verschure, ICREA Research Professor and Group Leader at IBEC
Some presumed that no such mechanism existed in biological neural networks. However, based on a combination of considerations from the current practice of AI, computational neuroscience, and an analysis of the hippocampal physiology, with this new article the authors show that self-supervision and error backpropagation co-exists in the brain, and with a very specific area of the brain involved: the hippocampus.
The circuitry and anatomy of learning
The hippocampus, a structure in the vertebrate brain, has long been understood to play a crucial role in memory and learning. But key questions have remained unanswered: how does it know what, and when, to learn? And what is the mechanism driving this? “Signals from the external environment pass through several brain structures before arriving at the hippocampus — the final station of sensory processing”, explains Dr. Diogo Santos-Pata, first author of the article and postdoctoral researcher at SPECS lab. Being able to compare new signals with the memory they trigger enables the hippocampus to learn about changes in our environment; a prediction Paul Verschure had made in 1993 based on neural embodied network models.
More specifically, and thanks to the close interaction with the neurophysiologist Ivan Soltesz and his team at Stanford University, researchers show that the hippocampus contains a network of neurons that control neuronal signals and information in a similar manner to the operations of artificial neural networks underlying the current AI revolution.
“Our main finding was putting in perspective not only the circuitry and the anatomy of the hippocampal complex but also the types of neurons that drive learning and allow the hippocampus to be fully autonomous in deciding what and when to learn”, Santos-Pata points out.
“It’s especially interesting because self-supervised machine learning-driven by error backpropagation is currently gaining a lot of traction and attention in the world of artificial intelligence and this is the first study to provide a comprehensive biological explanation for this mechanism”, added Santos-Pata.
Reference article: Diogo Santos-Pata, Adrián F. Amil, Ivan Georgiev Raikov, César Rennó-Costa, Anna Mura, Ivan Soltesz, Paul F.M.J. Verschure. “Epistemic Autonomy: Self-supervised Learning in the Mammalian Hippocampus”. Trends in Cognitive Sciences, 2021.
More information: gorts@ibecbarcelona.eu